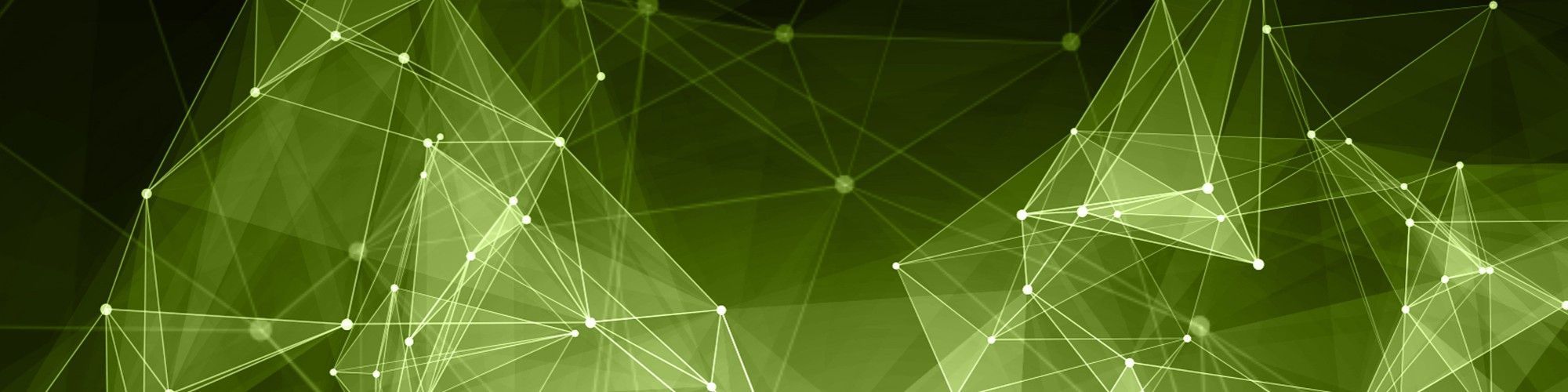
I am an Assistant Professor of Statistics (Tenure-track) at the Department of Economics and Finance, University of Rome Tor Vergata. Prior to my current position, I had a Research Associate position at the Vrije Universiteit Amsterdam and a PostDoc position at the University of Cyprus. I received the Ph.D. in Statistical Sciences from the University of Bologna. I have been awarded with the Award for the Best Ph.D. thesis in Statistics 2022 by the Italian Statistical Society. I also hold a Marie Skłodowska-Curie Individual Fellowship financed by the European Commission.
Research interests: Discrete-valued time series, Econometrics, Forecasting economic variables, Network time series, Observation-driven models, Time Series Analysis.
Download full CV here
Email: mirko.armillotta2@gmail.com
Research
Published Articles
- Armillotta M., Gorgi P. (2024)   Pseudo-variance quasi-maximum likelihood estimation of semi-parametric time series models.   Journal of Econometrics , accepted.
- Armillotta M., Tsagris M., and Fokianos K. (2024)   Inference for Network Count Time Series with the R Package PNAR.   The R Journal, 15(4): 255-269.
- Armillotta M., Fokianos K., and Guizzardi A. (2024)   Unveiling Venice's hotels competition networks from dynamic pricing digital market.   Journal of the Royal Statistical Society Series A: Statistics in Society, 187(1): 130-157.
- Armillotta M., Fokianos K. (2023)   Nonlinear Network Autoregression.   The Annals of Statistics, 51(6): 2526-2552.   [Open access version]
- Armillotta M., Fokianos K. (2023)   Count Network Autoregression.   Journal of Time Series Analysis, 45(4): 584-612.
- Armillotta M., Luati A. and Lupparelli M. (2022)   Observation-driven models for discrete-valued time series.   Electronic Journal of Statistics 16(1): 1393-1433.
Other Pubblications
- Armillotta M. (2023)   Two-stage weighted least squares estimator of multivariate conditional mean observation-driven time series models.   Book of short Papers SIS 2023, Pearson, pp. 770-775.
- Armillotta M., Luati A. and Lupparelli M. (2023)   An overview of ARMA-like models for count and binary data.   Trends and Challenges in Categorical Data Analysis, Springer, 2023, pp. 233-274.
- Armillotta M., Fokianos K., and Krikidis I. (2023)   Bootstrapping Network Autoregressive Models for Testing Linearity.   Data Science in Applications, Springer, 2023, pp. 99-116.
- Armillotta M., Fokianos K. and Krikidis I. (2022)   Generalized Linear Models Network Autoregression.   Network Science, Springer, 2022, pp. 112-125.
- Armillotta M., Luati A. and Lupparelli M. (2020)   Observation-driven models for storm counts.   Book of short Papers SIS 2020, Pearson, 2020, pp. 863-868.
- Armillotta M., Luati A. and Lupparelli M. (2019)   Stationarity of a general class of observation driven models for discrete valued processes.   Book of short Papers SIS 2019, Pearson, 2019, pp. 31-39.
Working Papers
- Armillotta M. (2023)   Two-stage weighted least squares estimator of multivariate discrete-valued observation-driven models, under review.
Software
- Tsagris M., Armillotta M. and Fokianos K.   R Package PNAR: Poisson Network Autoregressive Models.  
Curriculum Vitae
Download full CV here
Current Position
- 2024 - present, Assistant Professor of Statistics (Tenure-track), Department of Economics and Finance, University of Rome Tor Vergata.
Past Positions
- 2022 - 2024, Research Associate, Department Econometrics and Data Science, Vrije Universiteit Amsterdam.
- 2023 - 2024, Candidate Fellow, Tinbergen Institute.
- 2020 - 2022, Postdoctoral Researcher, Department of Mathematics and Statistics, University of Cyprus.
- Feb 2020 - May 2020, Visiting Researcher, Department of Mathematics and Statistics, Lancaster University.
- 2017 - 2019, Teaching tutor, Department of Statistics and Department of Economics, University of Bologna.
Education
- 2017 - 2021, Ph.D. in Statistical Sciences, University of Bologna.   
Dissertation title: Essays on discrete valued time series models. - 2015 - 2017, M.A. (cum laude) in Statistics, Economics and Business, University of Bologna.
- 2012 - 2015, B.Sc. (cum laude) in Statistical Sciences, University of Bologna.
Teaching
- 2023 - 2024, Statistics PM. Teacher and Course coordinator, Vrije Universiteit Amsterdam (English).
- 2022 - 2023, Econometrics II. Teacher, Vrije Universiteit Amsterdam (English).
Awards & Fellowships
- Marie Skłodowska-Curie Individual Fellowship financed by the European Commission (2023-present).
- Award for the Best Ph.D. thesis in Statistics 2022, awarded by the Italian Statistical Society.